Chatbot Analytics 2025: 10 Metrics for Higher Performance
Chatbot analytics provide key insights into how your chatbot is performing, how users interact with it, and where improvements are needed to boost its effectiveness.
In this guide, we’ll explore the 10 core metrics that help you evaluate your AI chatbot’s performance.
Whether you’re looking to refine your chatbot's user engagement or measure ROI, these insights will give you the knowledge you need to succeed.
What Is Chatbot Analytics?
Chatbot analytics is an essential tool that gives you deep insights into your chatbot’s performance.
By analyzing data from user interactions, you can see what’s working well, identify gaps, and make informed decisions to enhance your chatbot’s capabilities.
When you focus on the metrics of a chatbot that matter, you’re equipped to refine its functions, improve user experiences on your website, and align its performance with your business objectives.
Why Are Chatbot Analytics Important?
Why Are Chatbot Analytics Important?
With the increasing use of chatbots in customer service, understanding their performance is crucial. According to Intercom, 74% of consumers expect to chat with bots and appreciate their 24/7 availability.
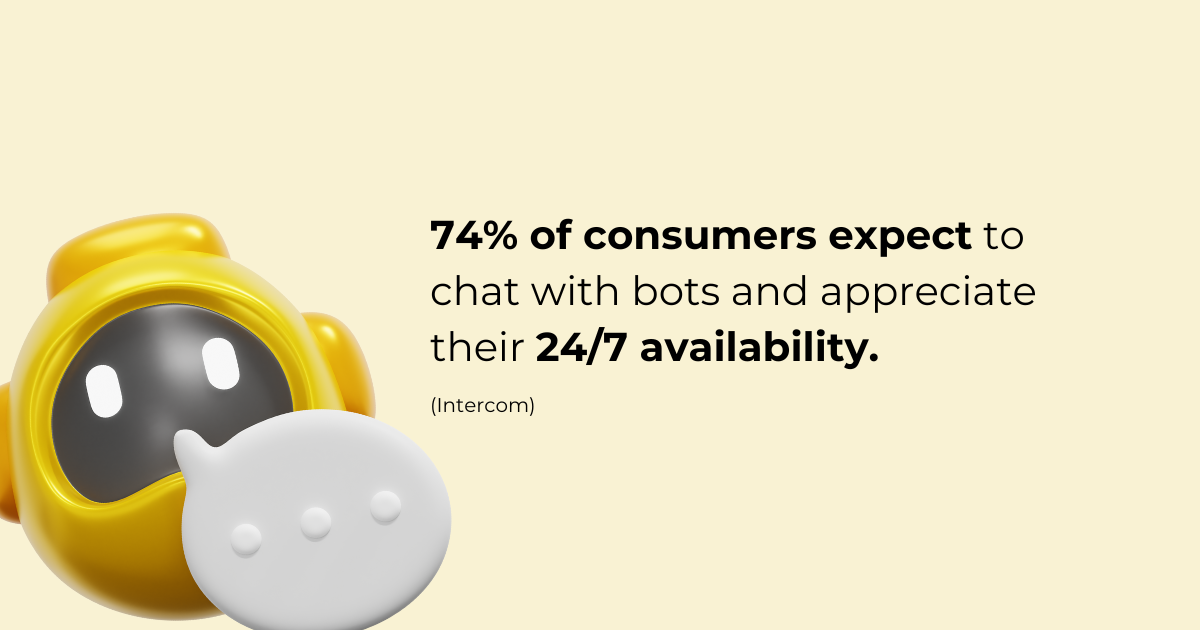
This high expectation underscores ensuring your chatbot effectively meets user needs.
Chatbot analytics enables businesses to monitor and improve chatbot performance, leading to enhanced customer satisfaction and loyalty.
1. Optimizing the Customer Experience
Chatbot analytics allow you to evaluate the chatbot's real-time performance. Tracking the resolution rate—the percentage of conversations successfully concluded—helps gauge the chatbot's efficiency in addressing user queries.
For instance, a Gartner survey found that chatbots resolve 58% of returns and cancellations but only 17% of billing disputes.
By analyzing these rates, you can identify areas where the chatbot excels and where it needs improvement, ensuring it consistently meets user expectations.
2. Enhancing Operational Efficiency
Businesses invest in chatbots to handle large volumes of interactions without overloading human support teams. Chatbot analytics help measure how effectively the bot fulfills this purpose.
If the human support switch rate is higher than expected, it's a sign that the chatbot needs further training in handling specific questions.
By addressing these weaknesses, you can reduce unnecessary escalations, allowing human agents to focus on more complex tasks and ultimately improving overall productivity.
In fact, 67% of business leaders say bots increase sales, and chatbots can save about $300,000 a year in operational costs (Intercom).
3. Measuring ROI and Business Value
Understanding how to measure the success of a chatbot is crucial for determining its impact on the business. Metrics such as the goal completion rate (GCR) provide concrete data on whether the chatbot is meeting predefined objectives, such as processing orders or resolving service issues.
A high GCR indicates that the chatbot effectively guides users to completion, adding value to both the business and the user. Additionally, faster response times are correlated with higher user satisfaction and engagement.
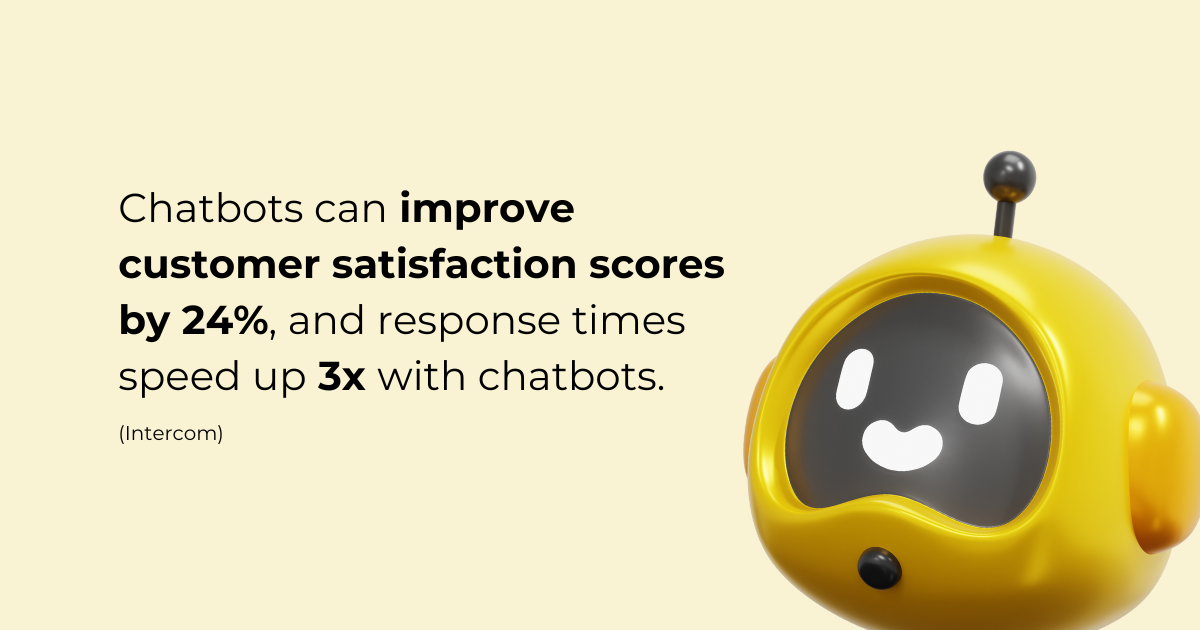
4. Identifying and Addressing Weaknesses
Monitoring missed utterances—instances where the chatbot fails to understand user input—provides actionable data on where improvements are needed.
High rates of missed utterances may indicate that the chatbot’s natural language processing capabilities require further training.
By analyzing these metrics, you can enhance the chatbot's understanding and responsiveness, leading to a smoother user experience.
5. Gaining Insights into User Behavior
Chatbot interactions offer valuable data to understand user preferences and behavior. Analyzing metrics such as messages per chat can reveal whether users find the information they need or get stuck in prolonged conversations.
If users engage in lengthy exchanges, it might indicate that responses need to be more concise or that additional information should be provided upfront. These insights empower you to tweak the chatbot’s content and conversational flow for more efficient interactions.
10 Essential Chatbot Metrics to Measure the Success of Your Chatbot
AI Agent Analytics Metrics
1. Total Conversations
The Total Conversations metric represents the number of times users have initiated interactions with your chatbot over a specific period. It's a fundamental part of chatbot analytics, giving you insight into overall user engagement.
For example, using the LiveChatAI chatbot, you can view your total conversions as follows.
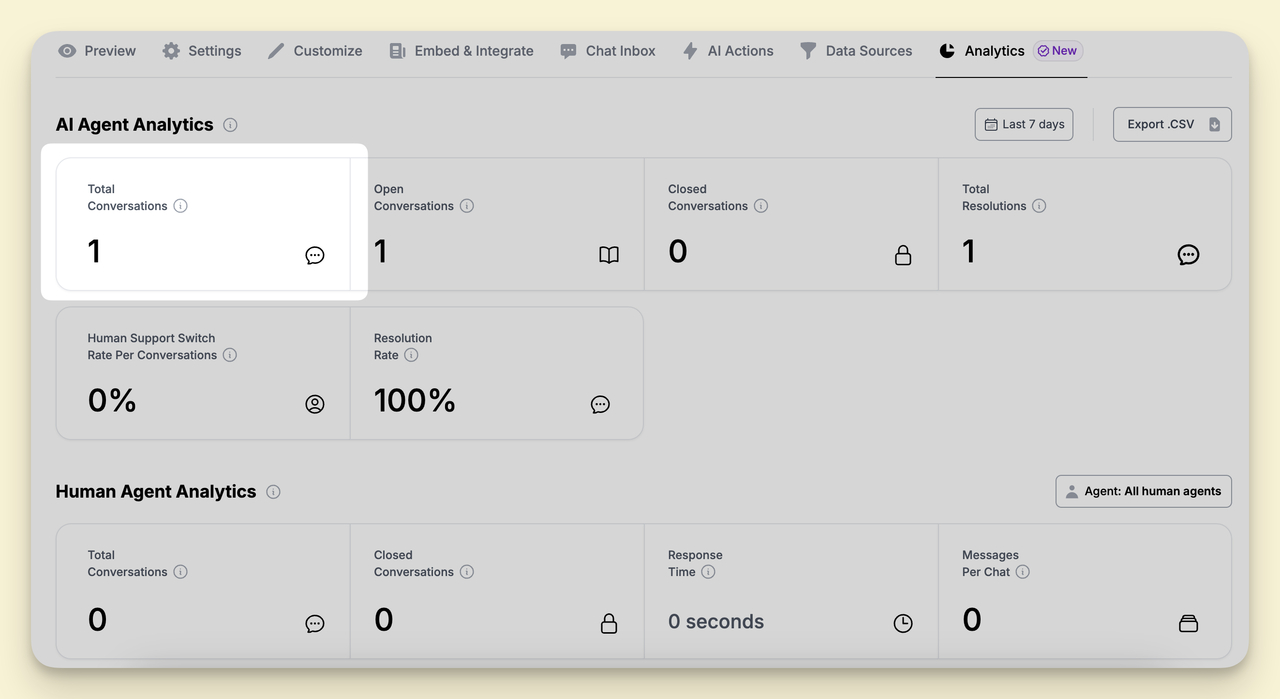
Why It Matters
- Gauge User Engagement: A higher number of conversations indicates that users are actively engaging with your chatbot.
- Measure Visibility: It reflects how easily users can find and access your chatbot on your website or app.
✅ High Total Conversations:
- What It Means: Your chatbot is visible and users are interested in interacting with it.
- Next Steps: Ensure these conversations are productive by guiding users toward desired actions like making a purchase or finding information.
❌ Low Total Conversations:
- What It Means: Users might not notice your chatbot, or they may not see its value.
- Next Steps: Improve your chatbot's visibility and make it more enticing to engage with.
Quick Tip: If you notice that conversations are high but not leading to conversions (like sales or sign-ups), focus on the quality of interactions. Ensure your chatbot is effectively guiding users toward your goals.
2. Open Conversations
The Open Conversations metric represents the number of chat sessions that are currently active and not yet resolved or closed.
In chatbot analytics, this metric helps you understand the real-time workload on your chatbot and customer support team.
For example, using the LiveChatAI chatbot, you can view your open conversions as follows.
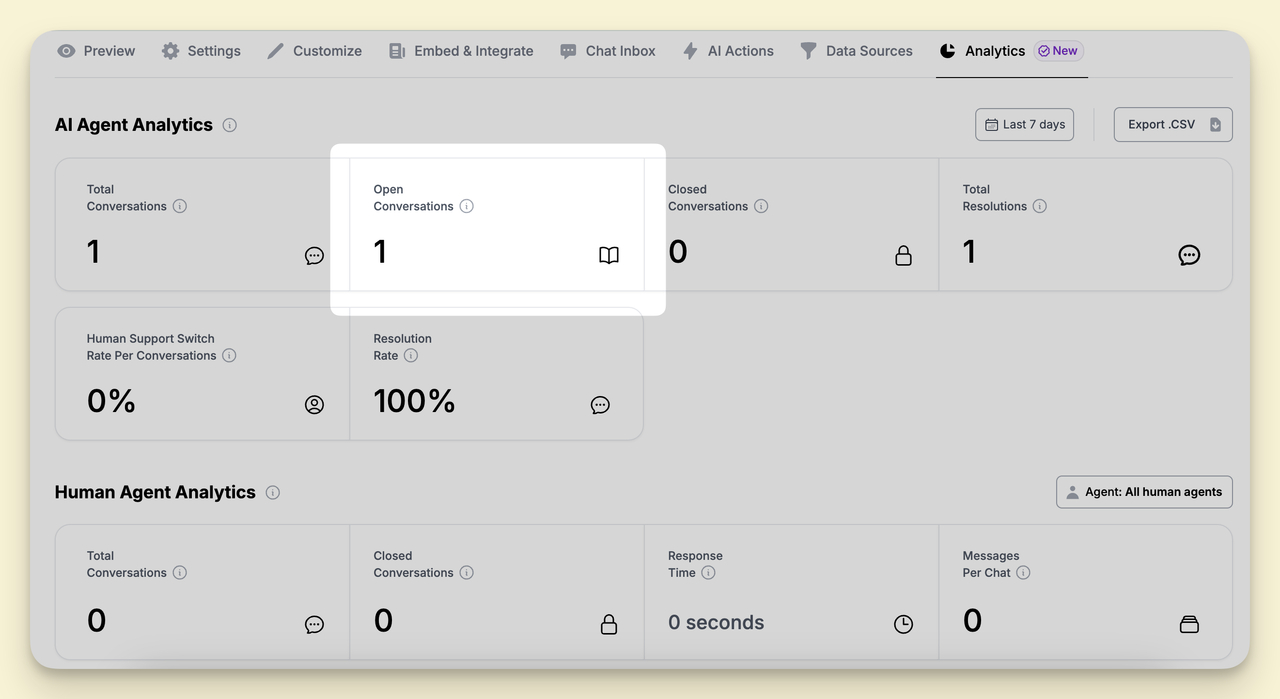
Why It Matters
- Resource Allocation: Knowing how many conversations are open at any given time helps you allocate resources efficiently, whether it's chatbot capacity or human agents.
- User Experience: A high number of open conversations might indicate users are waiting for responses, affecting satisfaction levels.
❗High Open Conversations:
- What It Means: There's high user engagement, but it could also signal a backlog if conversations aren't being resolved promptly.
- Next Steps: Address potential delays to improve user satisfaction.
❗Low Open Conversations:
- What It Means: Queries are being resolved quickly, or there may be low user engagement.
- Next Steps: Ensure that low numbers aren't due to technical issues or reduced traffic.
Quick tip: If open conversations are high, you can improve response times, improve decision trees, and implement pre-written answers for common queries. If low, make sure that your chatbot is working correctly and that the chatbot is providing useful answers.
3. Closed Conversations
Closed Conversations are interactions that have been concluded, either by the chatbot successfully resolving the user's query or by a human agent if the conversation was escalated.
For example, using the LiveChatAI chatbot, you can view your closed conversions as follows.
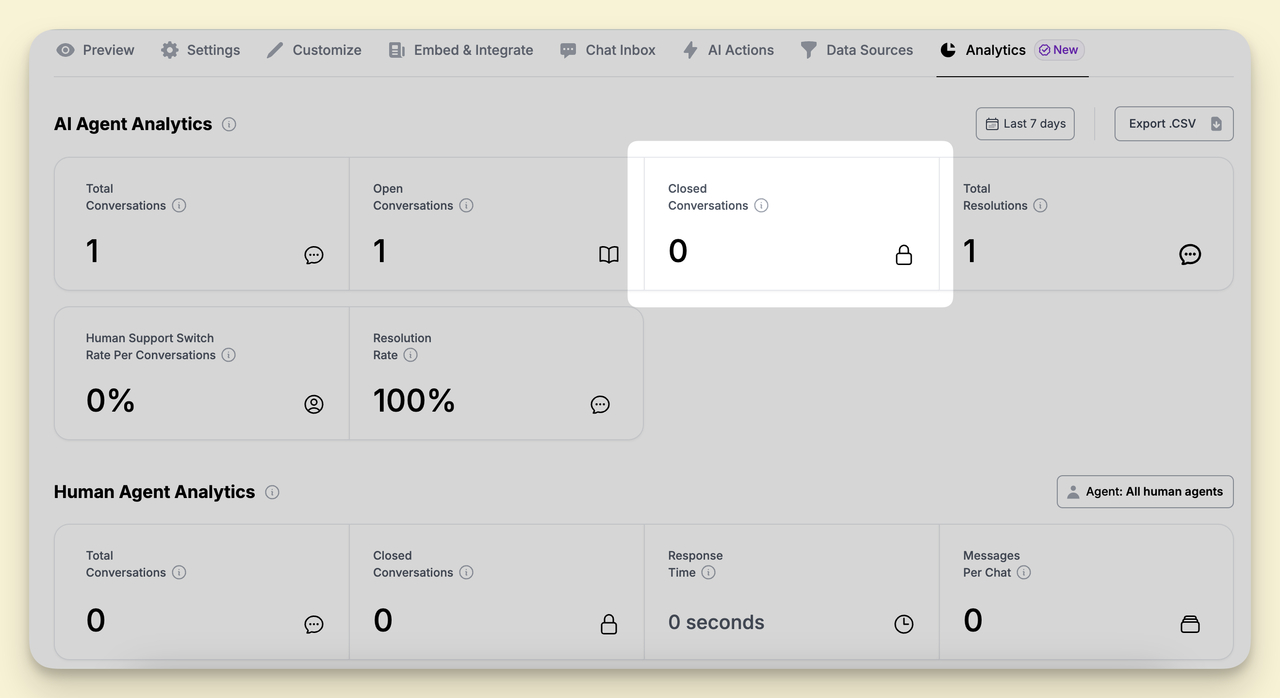
Why It Matters
- Measures Effectiveness: This metric shows how well your chatbot is performing in terms of resolving user issues.
- User Satisfaction: A higher number of closed conversations often correlates with higher user satisfaction.
✅ High Closed Conversations:
- What It Means: Your chatbot or support team is effectively resolving queries.
- Next Steps: Ensure quality remains high to maintain user satisfaction.
❌ Low Closed Conversations:
- What It Means: Users may be leaving chats without getting answers, or there may be issues preventing resolutions.
- Next Steps: Investigate and address obstacles to closing conversations.
Quick Tip: If you're noticing a high rate of closed conversations but low user satisfaction, take time to review the chat logs. Find where users are dissatisfied and assess if the chatbot's information is lacking or off-target. Finally, collect feedback to help enhance their experience.
4. Total Resolutions
The Total Resolutions metric counts the number of user issues successfully resolved by the chatbot without the need for human intervention.
For example, using the LiveChatAI chatbot, you can view your total resolutions as follows.

Why It Matters
- Efficiency Indicator: A high number of resolutions signifies that your chatbot effectively handles user queries, reducing the workload on human agents.
- Cost Savings: Efficient chatbots can lower operational costs by minimizing the need for additional staff.
✅ High Total Resolutions:
- What It Means: Your chatbot is performing well and effectively addressing user needs.
- Next Steps: Continue to maintain and update the chatbot to handle more complex queries.
❌ Low Total Resolutions:
- What It Means: Users often require human assistance, indicating limitations in your chatbot's capabilities.
- Next Steps: Enhance the chatbot to improve its problem-solving abilities.
Example Scenario: Suppose your chatbot analytics reveal that many users are asking about order tracking, but the chatbot isn't providing satisfactory answers. What can you do?
- Integrate the retail chatbot with your order management system.
- Enable the chatbot to provide real-time tracking information.
- Test the new feature to ensure it works smoothly.
5. Human Support Switch Rate per Conversation
The Human Support Switch Rate per Conversation measures the percentage of chatbot interactions where users request or are transferred to a human agent.
This is a crucial metric in chatbot analytics as it indicates how often your chatbot is unable to resolve issues independently.
For example, using the LiveChatAI chatbot, you can view your Human Support Switch Rate per Conversation as follows.
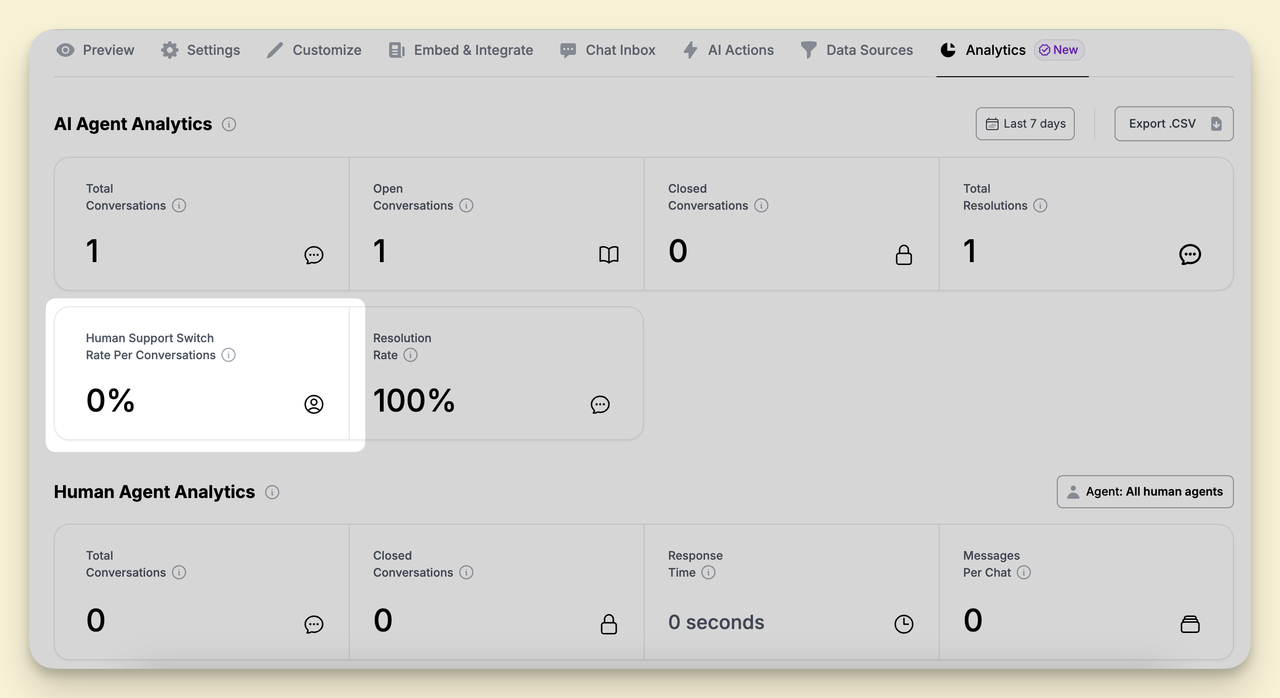
Why It Matters
- Assess Chatbot Effectiveness: A high switch rate may suggest that your chatbot isn't adequately addressing user needs.
- Resource Planning: Understanding this rate helps in allocating human support resources efficiently.
- Improve User Satisfaction: Minimizing unnecessary transfers can enhance the user experience.
❌ High Human Support Switch Rate:
- What It Means: Users frequently need human assistance, possibly due to chatbot limitations. However, AI chatbots can enhance human agents by handling routine inquiries and providing initial support.
- Next Steps: Investigate why users are seeking human help and improve chatbot capabilities.
✅ Low Human Support Switch Rate:
- What It Means: Your chatbot effectively handles user queries without needing to escalate.
- Next Steps: Continue monitoring to ensure quality remains high.
Quick Tip: You can reduce high switch rates by improving your chatbot's understanding, knowledge base chatbots, and user guidance. If the switch rate is low, ensure user satisfaction and easy access to human agents for an enhanced user experience.
6. Resolution Rate
The Resolution Rate is the percentage of conversations where the chatbot resolved the user's issue without the need for human intervention. It's a vital metric in chatbot analytics for assessing overall effectiveness.
For example, using the LiveChatAI chatbot, you can view your resolution rate as follows.

Why It Matters
- Efficiency Indicator: A high resolution rate means your chatbot efficiently handles user inquiries.
- User Satisfaction: Users prefer quick solutions without being passed around.
- Cost Savings: Higher resolution rates can reduce the need for human agents, lowering operational costs.
✅ High Resolution Rate:
- What It Means: Your chatbot effectively resolves most user issues.
- Next Steps: Maintain and fine-tune to handle even more complex queries.
❌ Low Resolution Rate:
- What It Means: Users' issues often remain unresolved, requiring human assistance.
- Next Steps: Identify weaknesses in the chatbot's responses and improve them.
Example Scenario: If your chatbot analytics show a low resolution rate, users frequently ask for shipping information that the chatbot can't provide. So, what can you do?
- Integrate shipping databases so the chatbot can access real-time information.
- Update the chatbot's scripts to include common shipping inquiries.
- Ensure the chatbot can guide users through tracking their orders. If you're using Shopify, consider exploring how to build a Shopify chatbot specifically for tracking orders.
Human Agent Analytics Metrics
7. Total Conversations Handled by Human Agents
This metric counts the number of conversations managed by your human support team. It's essential in chatbot analytics to understand how much workload is being handled by people versus the chatbot.
For example, using the LiveChatAI chatbot, you can view your total conversions handled by human agents as follows.

Why It Matters
- Resource Management: Helps in planning staffing needs and scheduling.
- Chatbot Effectiveness: A high number may indicate limitations in your chatbot's capabilities.
❌ High Total Conversations by Human Agents:
- What It Means: Your human agents are handling many queries, possibly due to the chatbot's inability to resolve issues.
- Next Steps: Identify common issues requiring human help and improve the chatbot accordingly.
✅ Low Total Conversations by Human Agents:
- What It Means: The chatbot is effectively handling most queries.
- Next Steps: Ensure the quality of chatbot interactions remains high to maintain user satisfaction.
8. Closed Conversations by Human Agents
This metric refers to the number of conversations that human agents have concluded. It provides insight into the efficiency and effectiveness of your human support team within your chatbot analytics.
For example, using the LiveChatAI chatbot, you can view your closed conversions handled by human agents as follows.

Why It Matters
- Performance Indicator: Shows how well human agents are resolving issues.
- Customer Satisfaction: Efficient closures generally lead to happier customers.
- Operational Efficiency: Helps in assessing the productivity of your support team.
✅ High Number of Closed Chats:
- What It Means: Human agents are efficiently resolving and closing conversations.
- Next Steps: Continue to support agents with training and resources.
❌ Low Number of Closed Chats:
- What It Means: Potential delays or difficulties in resolving customer issues.
- Next Steps: Investigate obstacles preventing agents from closing chats.
9. Response Time of Human Agents
The Response Time metric measures the average time it takes for human agents to respond to users after a conversation has been escalated from the chatbot. This is a critical component of your chatbot analytics because it directly affects user satisfaction and the overall efficiency of your customer support.
For example, using the LiveChatAI chatbot, you can view the response time of human agents as follows.

Why It Matters
- Customer Satisfaction: Users expect quick responses. A slow response time can lead to frustration and a negative impression of your brand.
- Service Quality: Reflects the efficiency of your support team and their ability to manage workload.
- Operational Efficiency: Helps identify staffing needs and optimize resource allocation.
❌ High Response Time:
- What It Means: There are delays in customer support, possibly due to understaffing or inefficient processes.
- Next Steps: Investigate the causes of delays and implement strategies to reduce response times.
✅ Low Response Time:
- What It Means: Human agents are responding promptly, leading to higher customer satisfaction.
- Next Steps: Maintain current practices and look for opportunities to improve even further.
10. Messages Per Chat by Human Agents
Messages Per Chat refers to the average number of messages exchanged between users and human agents during a conversation. This metric provides insights into the efficiency and complexity of interactions within your chatbot analytics framework.
For example, using the LiveChatAI chatbot, you can view your messages per chat by human agents as follows.

Why It Matters
- Efficiency Indicator: A higher number of messages may suggest that issues are complex or that communication is not as effective as it could be.
- User Experience: Users generally prefer quick resolutions. Fewer messages can indicate a more straightforward and satisfying experience.
- Training and Resources: Helps identify if agents need additional support or resources to resolve queries more efficiently.
❌ High Messages Per Chat:
- What It Means: Conversations are lengthy, possibly due to complex issues or inefficient communication.
- Next Steps: Look into ways to streamline conversations and improve agent effectiveness.
✅ Low Messages Per Chat:
- What It Means: Issues may be resolved quickly, or agents might be rushing, potentially missing important details.
- Next Steps: Ensure that agents are thorough and that users feel their issues are fully addressed.
Quick Tip: If there are fewer messages per chat but issues still occur, ensure that agents provide comprehensive information and ask follow-up questions. Implement post-chat surveys to gather user feedback and adapt it to refine agent training and support materials.
Tips for Improving Chatbot Performance Based on Analytics
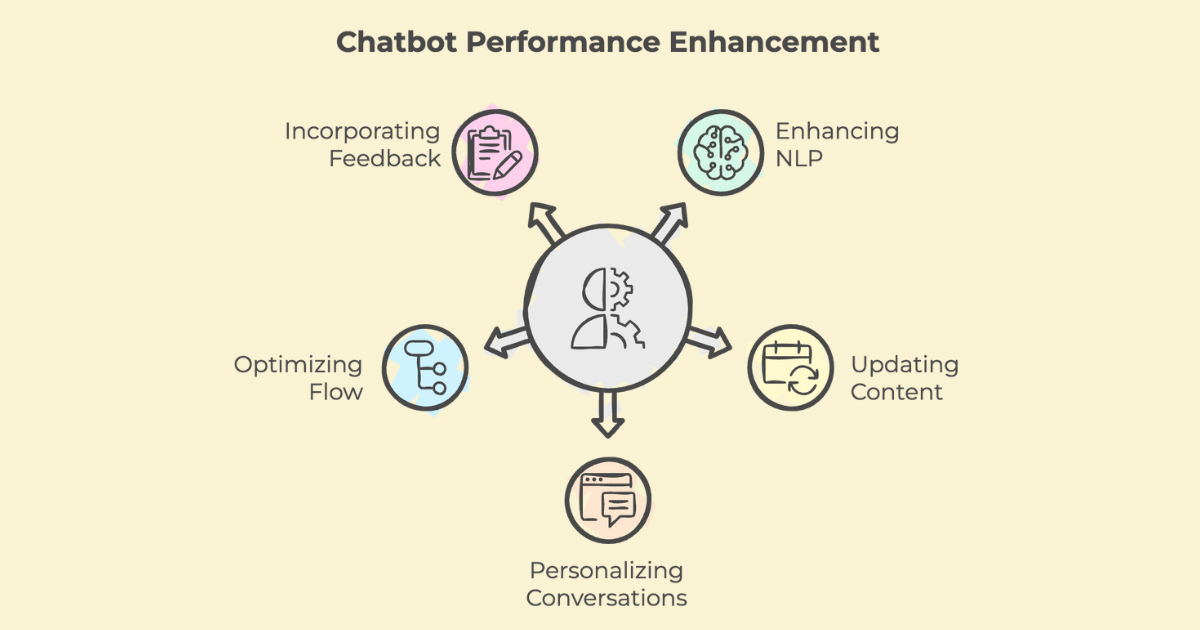
Enhancing NLP and AI Capabilities
- Regularly train the chatbot with diverse datasets.
- Implement machine learning for continuous improvement.
Regularly Updating Content and Responses
- Keep information current to maintain relevance.
- Update FAQs and scripted responses based on user queries.
Personalizing Conversations
- Use user data to tailor interactions.
- Implement personalization tokens and dynamic content.
Optimizing Conversation Flow
- Simplify navigation paths within the chatbot.
- A/B tests different conversation structures.
Incorporating User Feedback
- Encourage users to provide feedback.
- Use feedback to make targeted improvements.
Conclusion
Understanding and leveraging chatbot analytics is crucial for measuring the success of your chatbot and driving continuous improvement.
You can gain valuable insights into your chatbot's performance by closely monitoring key metrics such as total conversations, response time, user engagement rate, and customer satisfaction score (CSAT).
Implementing data-driven strategies based on these insights not only optimizes your chatbot's effectiveness but also elevates the overall customer experience.
Frequently Asked Questions (FAQs)
1. What Are the Key Metrics to Measure a Chatbot's Success?
Measuring a chatbot's success involves tracking several essential metrics:
- User Engagement Rate: Indicates how effectively your chatbot attracts and retains user attention.
- Resolution Rate: Measures the percentage of conversations the chatbot resolves without human intervention.
- Customer Satisfaction Score (CSAT): Reflects user satisfaction after interacting with your chatbot.
- Total Conversations: Shows overall user engagement and interest in your chatbot.
- Response Time: The speed at which your chatbot and human agents respond to user inquiries.
By monitoring these and other metrics discussed in this guide, you can gain a comprehensive understanding of your chatbot's performance.
2. How Do I Track My Chatbot's Performance?
Tracking your chatbot's performance can be achieved through:
- Built-in Analytics Dashboards: Many chatbot platforms offer analytics tools that provide real-time data on key metrics.
- Integration with Analytics Tools: Connect your chatbot to tools like Google Analytics for more in-depth insights.
- Regular Reporting: Schedule periodic reviews of your chatbot metrics to identify trends and make informed decisions.
- User Feedback: Collect feedback directly from users to supplement quantitative data with qualitative insights.
Utilize these methods to monitor your chatbot analytics effectively and make data-driven improvements.
3. What Is the Chatbot Containment Rate?
The Chatbot Containment Rate is a metric that measures the percentage of user interactions that are fully managed and resolved by the chatbot without the need for human intervention. A high containment rate indicates that your chatbot effectively handles queries, reducing the workload on human agents.
- Significance: A higher containment rate can lead to cost savings and increased efficiency.
- Improvement Strategies: Enhance your chatbot's knowledge base and natural language processing capabilities to improve this rate.
Monitoring the containment rate helps you understand your chatbot's effectiveness in providing autonomous support.
4. How Can I Improve My Chatbot's User Engagement?
Improving user engagement with your chatbot can be achieved through several strategies:
- Optimize Interaction Prompts: Use welcoming and friendly greetings to invite users to interact.
- Personalization: Tailor responses based on user data, previous interactions, or preferences.
- Interactive Elements: Incorporate quick reply buttons, images, or other multimedia elements to make interactions more engaging.
- A/B Testing: Experiment with different messages and prompts to see which ones resonate most with your audience.
By focusing on these areas, you can enhance user engagement and make your chatbot more appealing to users.
For further reading, you might be interested in the following: