Chatbot Containment Rate and Analytics That Matter
Chatbot Containment Rate (CCR) is a vital performance metric in the field of AI customer service that represents the capability of an AI-driven chatbot to efficiently manage and resolve customer queries independently without requiring human intervention.
The concept essentially gauges how well a chatbot 'contains' or confines the conversations within its response capabilities.
With growing customer preferences for self-service and round-the-clock service availability, the optimization of chatbot containment rates become even more critical in today's customer-centric approach to business.
What is the Chatbot Containment Rate?
The chatbot containment rate is a key performance metric that measures the percentage of customer interactions successfully handled by a chatbot without the need for human intervention.

This rate is crucial for businesses that utilize chatbots for customer service, support, or other interactive tasks.
A high containment rate indicates that the chatbot effectively resolves user queries autonomously, which can significantly enhance operational efficiency and reduce costs.
The containment rate is typically calculated by dividing the number of interactions fully resolved by the chatbot by the total number of interactions initiated with the chatbot, then multiplying the result by 100 to get a percentage.
The Importance of Measuring Chatbot Containment Rate
Measuring the chatbot containment rate is essential for several reasons.
Firstly, it provides insights into the effectiveness and efficiency of the chatbot. By analyzing this rate, organizations can assess whether their chatbot meets the needs of their customers or if improvements are necessary.
Secondly, a high containment rate can lead to reduced operational costs as fewer resources are needed for human agents who only step in for complex issues that the chatbot cannot handle.
Lastly, monitoring this metric helps improve customer satisfaction by ensuring that users receive quick and accurate responses to their inquiries, enhancing their overall experience with the service.
Businesses can improve both their customer service quality and operational productivity by optimizing the chatbot containment rate.
Factors Affecting Chatbot Containment Rate
Several factors can influence the containment rate of a chatbot, impacting its ability to resolve interactions without human intervention.

Understanding these factors can help in enhancing chatbot performance and customer satisfaction:
1. Chatbot Training and Knowledge Base 📚: The more comprehensive and up-to-date the chatbot's knowledge base, the better it is equipped to handle a wide range of queries. Regular training with new information and feedback is crucial for maintaining a high containment rate.
2. Natural Language Processing Capabilities 🗣️: A chatbot's ability to understand and process natural language effectively determines how well it can interpret user inquiries. Advanced NLP can discern nuances in language, making the chatbot more effective.
3. User Query Complexity 🤔: The complexity of users' questions significantly affects containment rates. Chatbots are more likely to resolve simple queries, whereas more complex issues may require human assistance.
4. Chatbot Design and User Interface 👾: An intuitive and user-friendly interface helps users interact more effectively with the chatbot, leading to higher containment rates. Poorly designed interfaces can frustrate users, prompting them to seek human help.
5. Integration and Automation Capabilities 🔗: Chatbots integrated well with backend systems and can perform automated tasks (like booking appointments or processing orders) and resolve more interactions without human input.
6. User Expectations and Behavior 🧍: User adaptability and expectations can also influence containment rates. Users familiar with digital technologies and have realistic expectations about chatbot capabilities tend to have higher satisfaction and success rates in their interactions.
Organizations can strategically improve their chatbots by focusing on these factors, aiming for a higher containment rate and better overall efficiency.
How to Calculate Chatbot Containment Rate
Calculating the chatbot containment rate is straightforward but crucial for evaluating the effectiveness of your chatbot—something that might be especially relevant if you're exploring how different technologies like Firebase or React could integrate into a user engagement scenario, such as a coffee meetup website.
Here's how you can calculate this important metric:

The step-by-step calculation:
1. Track Total Interactions: Count all interactions that users start with the chatbot over a specific period (e.g., daily, weekly, monthly).
2. Identify Resolved Interactions: Determine how many of these interactions are resolved entirely by the chatbot without transferring to a human agent.
3. Apply the Formula: Divide the number of resolved interactions by the total number of interactions to find the containment rate. Multiply the result by 100 to convert it into a percentage.
For example, if your chatbot handles 200 interactions in a day and resolves 160 of them without human help, the containment rate would be (160/200)×100%=80%.
This means that 80% of user queries are completely managed by the chatbot, indicating a high level of efficiency and effectiveness in routine query handling.
Understanding and monitoring this metric can help you fine-tune your chatbot’s responses, ensuring it can handle more complex interactions, which is essential for maintaining user engagement and satisfaction in any tech-driven solution.
What is Considered a Good Chatbot Containment Rate?
Determining a "good" chatbot containment rate can vary depending on factors such as industry, the complexity of tasks the chatbot is designed for, and customer expectations.
However, a general benchmark often cited in the industry is around a 70% to 90% containment rate. Here's a breakdown of why this range is considered effective:
- Industry Standards: Different industries may have varying expectations for chatbot performance. For instance, in sectors like e-commerce or simple customer service, a higher containment rate (closer to 90%) is often more achievable and expected because many of the inquiries are routine. In contrast, industries dealing with more complex queries, like finance or health, might consider a lower rate (around 70%) as acceptable due to the necessity of human intervention for nuanced issues.
- Complexity of Tasks: Chatbots designed to handle straightforward tasks such as FAQs, booking appointments, or tracking orders can achieve higher containment rates. More complex tasks, like resolving technical issues or providing personalized advice, often require human intervention, lowering the containment rate.
- Customer Satisfaction: The ultimate goal of a chatbot isn't just to contain interactions but to resolve them to the customer's satisfaction. A good containment rate should, therefore, not compromise the quality of service. If customers feel their needs are being met effectively by the chatbot, even a slightly lower containment rate can still be considered successful.
- Continuous Improvement: A "good" rate should also reflect an organization’s commitment to continuously improving its chatbot’s capabilities. Over time, as the chatbot learns from interactions and develops further, the containment rate should ideally improve, reflecting better service to users.
Strategies to Improve Chatbot Containment Rate
Improving the containment rate of a chatbot involves enhancing its capabilities to handle more interactions completely autonomously while ensuring customer satisfaction remains high.

Here are some effective strategies to consider:
1. Enhance Natural Language Processing (NLP) Capabilities:
- Tip: Invest in advanced NLP tools that improve the chatbot’s understanding of user queries, including context, sentiment, and intent. This can be achieved through continuous training on a diverse set of data and regular updates to the NLP model.
2. Expand the Knowledge Base:
- Tip: Continuously update and expand the chatbot’s knowledge base to cover more topics and include up-to-date information. This should also involve incorporating feedback from unresolved queries to fill in the gaps in the chatbot's understanding.
3. Simplify the User Interface:
- Tip: Design a straightforward and intuitive chatbot interface. Ensure that it guides users on how to ask questions effectively and clarifies what the chatbot can do. This minimizes user frustration and increases the likelihood of successful interactions.
4. Implement Feedback Loops:
- Tip: Set up mechanisms for capturing user feedback directly through the chatbot interface. Use this feedback to identify areas where the chatbot struggles and to train it for better performance in future interactions.
5. Optimize Chatbot for Specific Tasks:
- Tip: Tailor the chatbot to perform specific tasks with high efficiency. Specialization can significantly improve the containment rate as the bot becomes proficient in certain domains or queries.
6. Use Predictive Analytics:
- Tip: Employ predictive analytics based on past interactions and context to anticipate user needs. This allows the chatbot to offer more relevant solutions quickly, potentially resolving issues before the user even fully articulates them.
7. Integrate with Backend Systems:
- Tip: Ensure that the chatbot is seamlessly integrated with backend systems. This allows it to perform tasks such as checking account details, initiating transactions, or updating records autonomously without human intervention.
8. Regularly Review Performance Metrics:
- Tip: Regularly analyze performance metrics beyond just the containment rate. Look at customer satisfaction scores, resolution times, and the nature of escalated issues to understand where improvements are needed.
Case Studies on Successful Chatbot Containment Rate Optimization
Here are three case studies that illustrate successful strategies for optimizing chatbot containment rates across different industries:
Case Study 1: Global Retail Chain
Background: S…, a global retail chain, implemented a chatbot to handle customer inquiries about product availability, store locations, and order tracking.
Challenge: The initial containment rate was only 55%, with many customers needing to speak to human agents for additional information or unresolved issues.
Strategy Implemented:
- Enhanced Knowledge Base: S… expanded the chatbot’s knowledge base to include real-time inventory updates and store-specific information.
- Integration with CRM: The chatbot was integrated with the company’s CRM system to provide personalized responses based on customer history.
Outcome: The containment rate increased to 85%. Customers reported higher satisfaction due to quicker responses and personalized interaction, leading to increased repeat online visits.
Case Study 2: Financial Services Provider
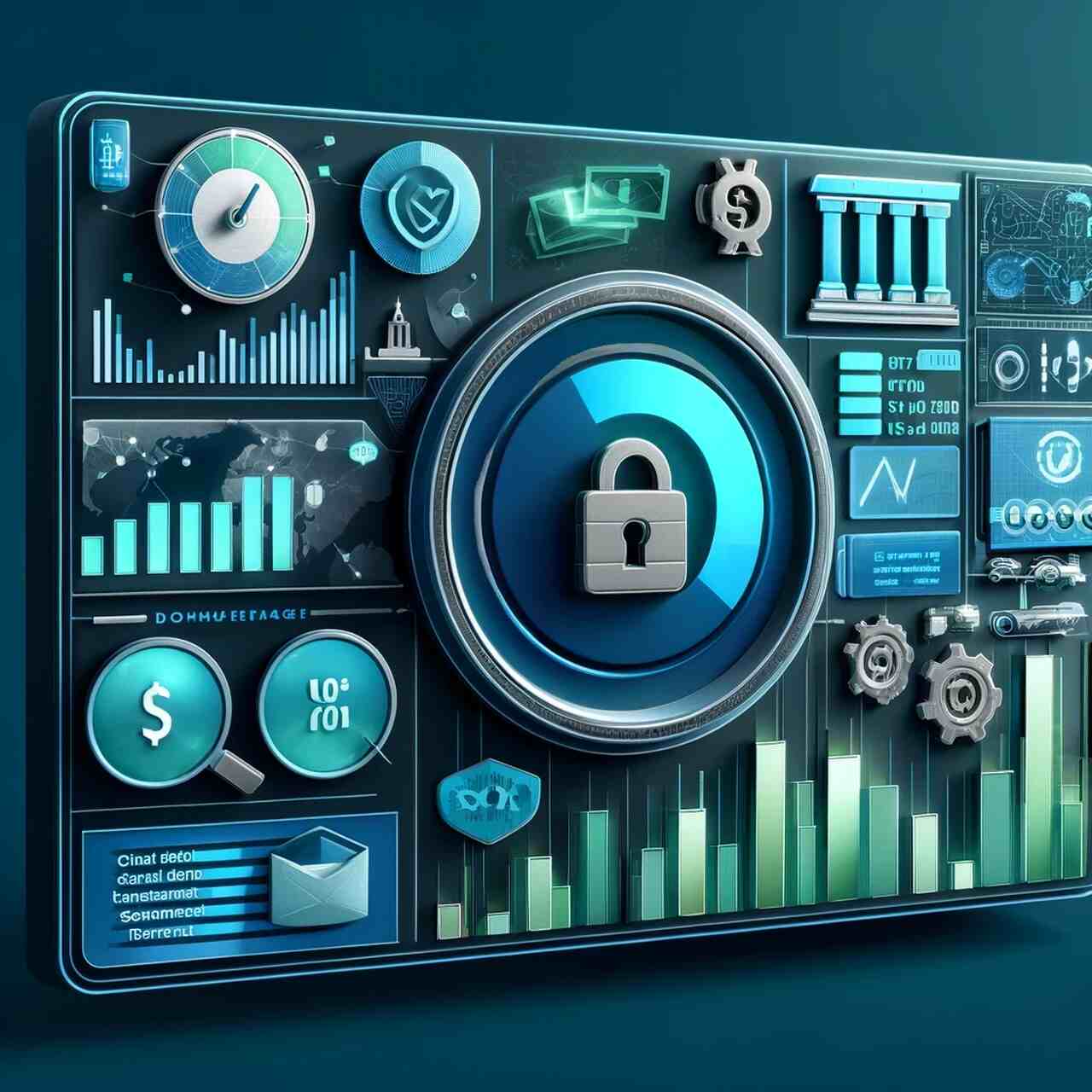
Background: F… deployed a chatbot to assist customers with common banking queries such as account balances, transaction histories, and credit card issues.
Challenge: The chatbot struggled with complex queries and compliance-related questions, resulting in a containment rate of just 60%.
Strategy Implemented:
- Advanced NLP Training: The chatbot underwent advanced training in natural language processing to better understand and respond to complex financial queries.
- Regulatory Compliance Module: A dedicated module for handling compliance and privacy-related queries was developed.
Outcome: F…’s chatbot containment rate improved to 78%. The chatbot's ability to handle complex and sensitive queries increased customer trust and reduced the workload on human agents.
Case Study 3: Healthcare Provider
Background: H… used a chatbot to schedule appointments, provide health tips, and triage initial patient inquiries.
Challenge: The chatbot had a low containment rate of 50% due to the complexity of medical queries and patients' need for detailed explanations.
Strategy Implemented:
- Patient Data Integration: The chatbot was integrated with patient medical records to provide personalized advice based on health history.
- Feedback Loop System: A feedback system was established where every chatbot session was followed up with a patient survey to gather insights and improve responses.
Outcome: The containment rate rose to 70%. Patients appreciated the personalized, informed interactions, and H… improved its operational efficiency while ensuring compliance with privacy regulations.
Conclusion
In sum, the containment rate of a chatbot provides essential insight into the bot's efficacy and the extent of its autonomous problem-solving capabilities.
While the direct implications of a high containment rate translate to efficiency and cost-effectiveness, it's important to remember that it's but one metric in the wider system of gauging a chatbot's overall performance.
Balancing a high containment rate with contributing factors will pave the way for superior AI functionality and enhanced customer service experiences for your business.
Frequently Asked Questions
Does a high containment rate mean the chatbot is more effective?
A high containment rate means the bot is able to handle more queries on its own, but effectiveness also involves other factors, such as correctly understanding user intent, offering helpful solutions, and providing a positive user experience.
How does the chatbot containment rate affect the customer experience?
A high containment rate means customers can receive quick, automated responses to their queries. However, if a chatbot can't handle more complex issues, it may impact customer experience negatively.
How does the containment rate factor into the overall evaluation of a chatbot's performance?
The containment rate is a key metric in analyzing a chatbot's effectiveness, but it should be looked at in conjunction with other metrics such as customer satisfaction scores (CSAT), first contact resolution (FCR), and the average handling time (AHT). This gives a broader perspective of the chatbot's proficiency.